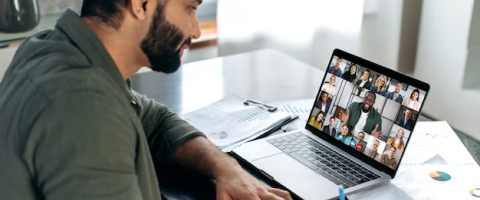
Expand your horizons beyond us by tapping into the wealth of knowledge from our peers and mentors
Each week, you ll have the exclusive opportunity to soak up valuable insights from experts who have left their mark in the industry.
Congratulations on being hired by Wipro : Shilpa - 5.8 LPA || Congratulations on being hired by TCS : Hemanth - 7.4 LPA || Congratulations on being hired by Infosys : Aruv - 8.2 LPA || Congratulations on being hired by Walmart : Abhishek - 4.2 LPA || Congratulations on being hired by Microsoft : Shreya - 6.4 LPA
Congratulations on being hired by Wipro : Shilpa - 5.8 LPA || Congratulations on being hired by TCS : Hemanth - 7.4 LPA || Congratulations on being hired by Infosys : Aruv - 8.2 LPA || Congratulations on being hired by Walmart : Abhishek - 4.2 LPA || Congratulations on being hired by Microsoft : Shreya - 6.4 LPA
Congratulations 🎉 for Amrutha Varshini to get placed in Avanthi Fellows, MNC with 20 Lacs package ||
Congratulations 🎉 for Vinod to get placed in MNC ALL STATE , Bangalore ||
Congratulations 🎉 for Maduri to get placed in to get placed in MNC WIPRO ||
Start working as Jr. Data science Intern in data science start-ups.
Gain Project Experience certificate from analytics Start-ups.
Hands on Industry experience with 1 to 1 client engagement and intensive training with practical real time projects.
Expand your horizons beyond us by tapping into the wealth of knowledge from our peers and mentors
Each week, you ll have the exclusive opportunity to soak up valuable insights from experts who have left their mark in the industry.
We strive to innovate when it comes to functionality. Our mission is to be the best, come and join the ride.
Real world projects offer invaluable benefits for students, fostering a practical learning environment that complements theoretical knowledge with hands on experience
Upon successful fulfillment of all course requirements and objectives, each student will be awarded a comprehensive Course Completion Certificate. This validates your commitment to learning and growth.
You ll receive a Project Experience Certificate, acknowledging your
hands on contribution and practical skills in project management.
We offer impactful internships to provide practical industry exposure, complementing academic learning. Additionally, our dedicated team provides comprehensive placement assistance, including resume workshops, interview preparation, and networking opportunities, to help students secure fulfilling roles in their chosen fields Rest assured, we are here for you.
We host live sessions to promptly address any questions you may have in real time.
Module-1 Learning Outcome :- ( Job Role :- Chat GPT Expert )
Description:
This Module is designed to equip participants with the knowledge and skills to effectively use R programming, Python and ChatGPT for data analysis tasks. Data analysis, traditionally a human-driven process, can greatly benefit from the integration of AI and natural language processing technologies. In this course, participants will explore how to leverage ChatGPT as a valuable assistant in data interpretation, report generation, and automating repetitive data-related tasks.
Target Audience:
Aspirants who wanted to become Data analysts, data scientists, business analysts, and professionals involved in data analysis, who want to incorporate AI technologies, particularly ChatGPT, into their workflows to improve efficiency, generate insights, and enhance data-driven decision-making.
Introduction to R programming
Introduction to Python Programming
Introduction to Python Libraries for Data Analysis
Data Analysis and ChatGPT
Data Collection and Preprocessing
Exploratory Data Analysis (EDA)
Statistical Analysis and Hypothesis Testing
Data Modeling and Machine Learning
Advanced Data Analysis Techniques
Integrating ChatGPT for Data Interpretation
ChatGPT in Industry-Specific Data Analysis
Advanced Applications with ChatGPT
Module-2 Learning Outcomes: – ( Job Role :- Asso Data Scientist / Machine Learning Expert )
Introduction to Data Science.
Different Disciplines of Data Science:
Why Machine Learning is the Future
Statistics & Probability
Introduction to Python
Deep Dive – Functions, OOPs, Modules, Errors, and Exceptions
Python in Data Manipulation
Sequences and File Operations
Introduction to NumPy, Pandas and Matplotlib
Introduction to Machine Learning with Python
Supervised Learning – I & II , Dimensionality Reduction
Unsupervised Learning
Association Rules Mining and Recommendation Systems
Reinforcement Learning
Time Series Analysis
Model Selection and Boosting
Cloud Computing & Deployment of Machine Learning Modals to Cloud
Module-3 Learning Outcome :- ( Job Role :- Data Science Project Manager )
Data Science Project Management
Module-4 Learning Outcome:- ( Job Role :- NLP Expert )
Introduction to Natural Language Processing
Natural Language Processing in TensorFlow
First Step of NLP – Text Processin
Extracting Named Entities from Text
Feature Engineering for Text
Mastering the Art of Text Cleaning
Interpreting Patterns from Text – Topic Modelling
Understanding Text Classification
Introduction to Language Modelling in NLP
Sequence-to-Sequence Modelling
Advanced NLP Tools
Module-5 Learning Outcome :- ( Job Role :- DEEP LEARNING Expert )
Introduction to Deep Learning
Deep Learning for NLP
Recurrent Neural Networks
Module-6 Learning Outcome :- ( Job Role :- Generative AI Expert )
Utilizing machine learning for early disease detection and personalized health insights, revolutionizing proactive healthcare.
Data-driven solutions for efficient urban planning and resource allocation, fostering sustainable development in smart cities.
Advanced algorithms providing real-time financial market predictions, empowering investors with insightful foresight.
Harnessing data science to evaluate environmental factors and their effects, driving informed decisions for eco-friendly initiatives.
What